What Is An Analysis? It’s a detailed examination of something, often involving data, to understand its components, workings, and implications. At WHAT.EDU.VN, we help you dissect this complex topic with clear explanations and free answers to your questions, using analytical thinking, critical thinking and problem-solving skills. Discover how analyses drive decision-making and innovation.
1. Decoding “What is an Analysis”: A Comprehensive Overview
An analysis is a systematic process of breaking down a complex topic or entity into smaller parts to gain a better understanding of it. This process involves careful observation, data collection, and the application of various analytical techniques. The goal is to identify patterns, relationships, and insights that would not be apparent through a simple overview. It’s about using analytical methods to extract meaningful information.
Alt text: Data analyst examining charts and graphs on a computer screen, signifying detailed examination and analytical processes.
2. The Core Components of an Analysis
Every robust analysis comprises several key elements that work together to deliver comprehensive insights. These elements include:
- Data Collection: Gathering relevant information from various sources.
- Data Processing: Cleaning, organizing, and transforming raw data into a usable format.
- Data Analysis: Applying statistical, mathematical, or qualitative methods to identify patterns and trends.
- Interpretation: Drawing conclusions and explaining the significance of the findings.
- Reporting: Presenting the results in a clear, concise, and understandable manner.
3. Why is Analysis Important?
Analysis is crucial because it transforms raw data into actionable intelligence. It empowers us to make informed decisions, solve complex problems, and predict future trends. Without analysis, we are left with fragmented information and guesswork.
4. Different Types of Analysis
The realm of analysis is vast, encompassing various types suited to different fields and purposes. Some common types include:
- Statistical Analysis: Using statistical methods to quantify relationships and test hypotheses.
- Qualitative Analysis: Exploring non-numerical data, such as interviews or texts, to understand meanings and perspectives.
- Financial Analysis: Evaluating financial performance and making investment decisions.
- Business Analysis: Identifying business needs and recommending solutions.
- Data Analysis: Examining large datasets to uncover hidden patterns and insights.
- Risk Analysis: Assessing potential risks and developing mitigation strategies.
- Gap Analysis: Comparing actual performance with desired performance to identify areas for improvement.
5. Statistical Analysis in Detail
Statistical analysis involves collecting and scrutinizing a sample of data to find patterns and trends. It’s a cornerstone of research and decision-making in various fields, providing a quantitative basis for conclusions.
5.1. Descriptive Statistics
Descriptive statistics summarize and describe the main features of a dataset. Common measures include:
- Mean: The average value.
- Median: The middle value.
- Mode: The most frequent value.
- Standard Deviation: A measure of the spread of data around the mean.
- Variance: The square of the standard deviation.
5.2. Inferential Statistics
Inferential statistics use sample data to make inferences and generalizations about a larger population. Key techniques include:
- Hypothesis Testing: Testing specific claims or hypotheses about a population.
- Confidence Intervals: Estimating a range of values within which a population parameter is likely to fall.
- Regression Analysis: Modeling the relationship between two or more variables.
- ANOVA (Analysis of Variance): Comparing the means of two or more groups.
5.3. Regression Analysis Explained
Regression analysis is a powerful tool for understanding the relationships between variables. It allows you to predict the value of a dependent variable based on the values of one or more independent variables.
Alt text: Scatter plot with regression line depicting the relationship between variables, illustrating regression analysis.
- Linear Regression: Models a linear relationship between the variables.
- Multiple Regression: Extends linear regression to include multiple independent variables.
- Logistic Regression: Predicts the probability of a binary outcome (e.g., yes/no).
- Polynomial Regression: Models a non-linear relationship between the variables.
5.4. Hypothesis Testing: A Closer Look
Hypothesis testing is a fundamental method in statistical analysis used to validate or reject assumptions about a population based on sample data. Here’s how it generally works:
-
Formulate Hypotheses:
- Null Hypothesis (H0): This is a statement of no effect or no difference. It’s what you aim to disprove.
- Alternative Hypothesis (H1): This states what you believe to be true if the null hypothesis is false.
-
Choose a Significance Level (α): This is the probability of rejecting the null hypothesis when it is actually true (Type I error). Common values are 0.05 (5%) or 0.01 (1%).
-
Calculate a Test Statistic: Based on your data and the type of test, compute a test statistic (e.g., t-statistic, z-statistic).
-
Determine the p-value: The p-value is the probability of observing a test statistic as extreme as, or more extreme than, the one computed, assuming the null hypothesis is true.
-
Make a Decision:
- If the p-value is less than or equal to the significance level (p ≤ α), reject the null hypothesis in favor of the alternative hypothesis.
- If the p-value is greater than the significance level (p > α), fail to reject the null hypothesis.
6. Qualitative Analysis: Exploring the “Why”
Qualitative analysis focuses on understanding the qualities, characteristics, and meanings behind phenomena. It is often used in social sciences, humanities, and market research to explore complex issues and gain deeper insights.
6.1. Common Qualitative Methods
- Interviews: Conducting structured or unstructured conversations to gather in-depth perspectives.
- Focus Groups: Facilitating group discussions to explore shared experiences and opinions.
- Content Analysis: Analyzing texts, images, or other media to identify patterns and themes.
- Ethnography: Immersing oneself in a cultural setting to understand behaviors and interactions.
- Case Studies: Conducting in-depth investigations of specific cases or events.
6.2. Thematic Analysis: Finding Patterns in Qualitative Data
Thematic analysis is a method for identifying, analyzing, and reporting patterns (themes) within qualitative data. It involves the following steps:
- Familiarization: Immersing yourself in the data by reading and re-reading it.
- Coding: Generating initial codes to identify interesting features of the data.
- Searching for Themes: Grouping codes into broader themes.
- Reviewing Themes: Refining and developing themes further.
- Defining and Naming Themes: Clearly defining the essence of each theme.
- Writing Up: Producing a narrative that weaves together the themes and provides evidence from the data.
6.3. Grounded Theory: Building Theories from Data
Grounded theory is a systematic methodology for developing theories based on data. Unlike traditional research where hypotheses are tested, grounded theory aims to generate new theories from the ground up.
- Data Collection: Start by collecting data through interviews, observations, or document analysis.
- Open Coding: Break down the data into discrete parts and identify initial codes.
- Axial Coding: Group codes into categories and identify relationships between them.
- Selective Coding: Select a core category and relate all other categories to it.
- Theory Development: Develop a theoretical framework based on the relationships between categories.
- Constant Comparison: Continuously compare new data with existing codes and categories to refine the theory.
7. Financial Analysis: Making Sense of Numbers
Financial analysis is the process of evaluating financial performance, assessing investment opportunities, and making informed financial decisions. It involves analyzing financial statements, ratios, and other relevant data.
7.1. Key Financial Statements
- Income Statement: Reports a company’s financial performance over a period of time.
- Balance Sheet: Provides a snapshot of a company’s assets, liabilities, and equity at a specific point in time.
- Cash Flow Statement: Tracks the movement of cash into and out of a company over a period of time.
7.2. Important Financial Ratios
- Liquidity Ratios: Measure a company’s ability to meet its short-term obligations.
- Current Ratio: Current Assets / Current Liabilities
- Quick Ratio: (Current Assets – Inventory) / Current Liabilities
- Profitability Ratios: Assess a company’s ability to generate profits.
- Gross Profit Margin: (Revenue – Cost of Goods Sold) / Revenue
- Net Profit Margin: Net Income / Revenue
- Solvency Ratios: Evaluate a company’s ability to meet its long-term obligations.
- Debt-to-Equity Ratio: Total Debt / Total Equity
- Efficiency Ratios: Measure how efficiently a company uses its assets.
- Inventory Turnover: Cost of Goods Sold / Average Inventory
- Accounts Receivable Turnover: Revenue / Average Accounts Receivable
7.3. Investment Analysis: Making Smart Choices
Investment analysis involves evaluating the potential returns and risks of different investment opportunities. This can include stocks, bonds, real estate, and other assets.
- Fundamental Analysis: Examining a company’s financial statements, industry trends, and competitive landscape to assess its intrinsic value.
- Technical Analysis: Using historical price and volume data to identify patterns and predict future price movements.
- Portfolio Management: Constructing and managing a portfolio of investments to achieve specific financial goals.
8. Business Analysis: Bridging the Gap
Business analysis is the practice of identifying business needs, recommending solutions, and facilitating change. Business analysts work as a liaison between business stakeholders and IT teams to ensure that projects align with business objectives.
8.1. Core Business Analysis Tasks
- Requirements Elicitation: Gathering requirements from stakeholders through interviews, workshops, and surveys.
- Requirements Analysis: Analyzing and documenting requirements in a clear and concise manner.
- Solution Design: Developing potential solutions to address business needs.
- Stakeholder Management: Managing relationships with stakeholders and ensuring their needs are met.
- Process Improvement: Identifying opportunities to improve business processes and increase efficiency.
8.2. SWOT Analysis: Understanding Strengths, Weaknesses, Opportunities, and Threats
SWOT analysis is a strategic planning tool used to evaluate the Strengths, Weaknesses, Opportunities, and Threats involved in a project or business venture.
- Strengths: Internal factors that give an organization an advantage.
- Weaknesses: Internal factors that put an organization at a disadvantage.
- Opportunities: External factors that could benefit an organization.
- Threats: External factors that could harm an organization.
8.3. Use Case Analysis: Mapping Out Interactions
Use case analysis is a technique used to describe the interactions between a user and a system. It helps to define the functionality of a system and ensure that it meets the needs of its users.
- Identify Actors: Determine who or what will interact with the system.
- Define Use Cases: Describe the specific interactions that actors will have with the system.
- Create Use Case Diagrams: Visually represent the relationships between actors and use cases.
- Write Use Case Descriptions: Provide detailed descriptions of each use case, including preconditions, postconditions, and alternative scenarios.
9. Data Analysis: Uncovering Insights
Data analysis is the process of inspecting, cleaning, transforming, and modeling data to discover useful information, draw conclusions, and support decision-making.
9.1. The Data Analysis Process
- Data Collection: Gathering data from various sources, such as databases, spreadsheets, and web APIs.
- Data Cleaning: Identifying and correcting errors, inconsistencies, and missing values in the data.
- Data Transformation: Transforming the data into a format that is suitable for analysis.
- Data Modeling: Applying statistical and machine learning techniques to identify patterns and relationships in the data.
- Data Visualization: Creating charts, graphs, and other visual representations of the data to communicate insights.
9.2. Key Data Analysis Techniques
- Data Mining: Discovering patterns and relationships in large datasets.
- Machine Learning: Using algorithms to learn from data and make predictions.
- Predictive Analytics: Forecasting future outcomes based on historical data.
- Text Analytics: Analyzing unstructured text data to extract insights.
- Web Analytics: Measuring and analyzing website traffic and user behavior.
9.3. Data Visualization: Telling Stories with Data
Data visualization is the graphical representation of information and data. By using visual elements like charts, graphs, and maps, data visualization tools provide an accessible way to see and understand trends, outliers, and patterns in data.
Alt text: Data visualization dashboard showcasing bar charts, pie charts, and line graphs, illustrating data communication.
- Bar Charts: Compare values across different categories.
- Line Graphs: Show trends over time.
- Pie Charts: Display proportions of a whole.
- Scatter Plots: Show the relationship between two variables.
- Heatmaps: Display the magnitude of a phenomenon as color in two dimensions.
10. Risk Analysis: Identifying Potential Problems
Risk analysis is the process of identifying, assessing, and mitigating potential risks. It is used in various fields, such as project management, finance, and healthcare, to minimize the likelihood and impact of adverse events.
10.1. The Risk Analysis Process
- Risk Identification: Identifying potential risks that could affect a project or organization.
- Risk Assessment: Evaluating the likelihood and impact of each risk.
- Risk Response Planning: Developing strategies to mitigate or avoid risks.
- Risk Monitoring and Control: Tracking and managing risks throughout the project lifecycle.
10.2. Risk Assessment Techniques
- Qualitative Risk Analysis: Assessing risks based on subjective judgments and expert opinions.
- Quantitative Risk Analysis: Using statistical and mathematical models to quantify the likelihood and impact of risks.
- Monte Carlo Simulation: Using random sampling to simulate potential outcomes and assess the range of possible results.
10.3. Risk Mitigation Strategies
- Risk Avoidance: Eliminating the risk altogether.
- Risk Reduction: Reducing the likelihood or impact of the risk.
- Risk Transfer: Transferring the risk to another party, such as through insurance.
- Risk Acceptance: Accepting the risk and preparing to deal with the consequences.
11. Gap Analysis: Identifying Discrepancies
Gap analysis is a technique used to compare actual performance with desired performance to identify areas for improvement. It helps organizations understand the “gap” between where they are and where they want to be.
11.1. The Gap Analysis Process
- Identify the Desired State: Define the goals and objectives that the organization wants to achieve.
- Assess the Current State: Evaluate the organization’s current performance and capabilities.
- Identify the Gaps: Compare the desired state with the current state to identify the gaps.
- Develop Action Plans: Create strategies and action plans to close the gaps.
- Implement and Monitor: Implement the action plans and monitor progress to ensure that the gaps are being closed.
11.2. Common Areas for Gap Analysis
- Performance Gap: Comparing actual performance with desired performance.
- Market Gap: Identifying unmet needs in the market.
- Skills Gap: Assessing the skills and competencies of employees.
- Technology Gap: Evaluating the organization’s technology infrastructure.
11.3. Benefits of Gap Analysis
- Identifies Areas for Improvement: Helps organizations focus their efforts on the areas that need the most attention.
- Improves Decision-Making: Provides a clear understanding of the gaps and the steps needed to close them.
- Enhances Strategic Planning: Supports the development of effective strategies and action plans.
- Increases Efficiency: Helps organizations use their resources more effectively.
12. The Role of Critical Thinking in Analysis
Critical thinking is an essential skill for anyone involved in analysis. It involves evaluating information, identifying assumptions, and forming well-reasoned conclusions.
12.1. Key Elements of Critical Thinking
- Analysis: Breaking down complex information into smaller parts.
- Interpretation: Understanding the meaning and significance of information.
- Inference: Drawing conclusions based on evidence and reasoning.
- Evaluation: Assessing the credibility and validity of information.
- Explanation: Clearly and logically communicating ideas and conclusions.
- Self-Regulation: Monitoring one’s own thinking processes and identifying areas for improvement.
12.2. Applying Critical Thinking to Data Analysis
- Question Assumptions: Challenge the assumptions that underlie the data and analysis.
- Evaluate Sources: Assess the credibility and reliability of the data sources.
- Consider Alternative Explanations: Explore different interpretations of the data.
- Identify Biases: Recognize and mitigate potential biases in the analysis.
- Draw Well-Reasoned Conclusions: Base conclusions on evidence and logical reasoning.
12.3. Enhancing Analytical Skills
Improving your analytical abilities involves cultivating several vital skills. These include:
- Active Listening: Paying close attention to understand the nuances of what is being communicated.
- Effective Communication: Conveying information clearly and concisely.
- Problem-Solving: Identifying and resolving complex issues using logical and creative approaches.
- Attention to Detail: Focusing on accuracy and thoroughness in all tasks.
- Continuous Learning: Staying updated with the latest knowledge and techniques in your field.
13. Tools Used in Analysis
Analysts rely on a variety of tools to collect, process, and analyze data. These tools range from simple spreadsheets to sophisticated statistical software packages.
13.1. Spreadsheet Software (e.g., Microsoft Excel, Google Sheets)
Spreadsheet software is widely used for data entry, organization, and basic analysis. It provides tools for creating charts, graphs, and performing simple statistical calculations.
13.2. Statistical Software (e.g., SPSS, SAS, R, Python)
Statistical software packages offer advanced analytical capabilities, including hypothesis testing, regression analysis, and data mining.
- SPSS (Statistical Package for the Social Sciences): A user-friendly statistical software package commonly used in social sciences and business research.
- SAS (Statistical Analysis System): A powerful statistical software package used for data management, advanced analytics, and business intelligence.
- R: A free and open-source programming language and software environment for statistical computing and graphics.
- Python: A versatile programming language with powerful libraries for data analysis, machine learning, and scientific computing (e.g., NumPy, Pandas, Scikit-learn).
13.3. Data Visualization Tools (e.g., Tableau, Power BI)
Data visualization tools help analysts create interactive charts, graphs, and dashboards to communicate insights effectively.
- Tableau: A popular data visualization tool that allows users to create interactive dashboards and visualizations from various data sources.
- Power BI: A business analytics tool by Microsoft that provides interactive visualizations and business intelligence capabilities.
13.4. Database Management Systems (e.g., SQL, MySQL)
Database management systems are used to store, manage, and retrieve large volumes of data.
- SQL (Structured Query Language): A standard language for managing and manipulating data in relational database management systems.
- MySQL: A popular open-source relational database management system.
14. Ethical Considerations in Analysis
Ethical considerations are paramount in analysis. Analysts must ensure that their work is objective, transparent, and unbiased.
14.1. Data Privacy and Security
Analysts must protect the privacy and security of data, especially when dealing with sensitive information.
- Anonymization: Removing or masking identifying information from data.
- Encryption: Encoding data to prevent unauthorized access.
- Data Governance Policies: Establishing policies and procedures for managing data responsibly.
14.2. Avoiding Bias and Misrepresentation
Analysts must be aware of potential biases in their data and analysis and take steps to mitigate them.
- Sampling Bias: Ensuring that the sample is representative of the population.
- Confirmation Bias: Avoiding the tendency to interpret data in a way that confirms pre-existing beliefs.
- Transparency: Clearly documenting the methods and assumptions used in the analysis.
14.3. Responsible Reporting
Analysts must present their findings in a clear, accurate, and unbiased manner.
- Contextualization: Providing sufficient context to understand the results.
- Limitations: Acknowledging the limitations of the analysis.
- Objectivity: Avoiding sensationalism or exaggeration.
15. The Future of Analysis
The field of analysis is constantly evolving, driven by technological advancements and the increasing availability of data.
15.1. Artificial Intelligence (AI) and Machine Learning (ML)
AI and ML are transforming the way we analyze data, enabling us to automate tasks, discover patterns, and make predictions with greater accuracy.
- Automated Data Analysis: Using AI to automate the process of data cleaning, transformation, and modeling.
- Predictive Analytics: Using ML to forecast future outcomes based on historical data.
- Natural Language Processing (NLP): Using AI to analyze and understand unstructured text data.
15.2. Big Data Analytics
Big data analytics involves analyzing large and complex datasets to uncover insights that would not be possible with traditional methods.
- Hadoop: An open-source framework for storing and processing large datasets.
- Spark: A fast and general-purpose cluster computing system for big data processing.
- Cloud Computing: Using cloud-based services to store and analyze big data.
15.3. The Democratization of Analysis
The democratization of analysis refers to the trend of making analytical tools and techniques more accessible to a wider audience.
- Self-Service Analytics: Empowering users to perform their own data analysis without the need for specialized skills.
- Citizen Data Scientists: Training employees to become data analysts within their respective domains.
- Data Literacy: Promoting data literacy throughout the organization to enable better decision-making.
16. Practical Applications of Analysis
Analysis is applied in numerous fields to enhance decision-making, optimize processes, and gain strategic advantages.
16.1. Healthcare
- Predictive Modeling: Anticipating patient outcomes and optimizing treatment plans.
- Healthcare Analytics: Enhancing healthcare delivery and minimizing costs.
- Epidemiological Analysis: Monitoring and managing disease outbreaks.
16.2. Marketing
- Customer Segmentation: Identifying distinct customer categories and customizing marketing strategies.
- Marketing Campaign Optimization: Assessing campaign effectiveness and maximizing ROI.
- Market Trend Analysis: Monitoring market developments and adapting to shifting consumer preferences.
16.3. Finance
- Risk Management: Assessing and alleviating financial risks.
- Fraud Detection: Detecting fraudulent activities and safeguarding assets.
- Investment Analysis: Assessing investment prospects and maximizing returns.
16.4. Education
- Student Performance Analysis: Monitoring student progress and pinpointing areas for enhancement.
- Curriculum Development: Tailoring curricula to align with student requirements.
- Educational Trend Analysis: Observing trends in education and implementing innovative strategies.
17. Learning Analysis: Resources and Opportunities
If you’re looking to deepen your understanding of analysis, numerous resources and opportunities are available.
17.1. Online Courses and Tutorials
- Coursera: Offers a variety of courses on data analysis, statistics, and related topics.
- edX: Provides courses from top universities on data science and analytics.
- Khan Academy: Offers free tutorials on statistics and data analysis.
- Udemy: Provides a variety of courses on data analysis, business analysis, and financial analysis.
17.2. Books and Publications
- “Data Analysis with Python” by Wes McKinney: A comprehensive guide to data analysis using Python.
- “Business Statistics” by David R. Anderson, Dennis J. Sweeney, and Thomas A. Williams: A comprehensive textbook on business statistics.
- “Financial Statement Analysis” by Krishna G. Palepu, Paul M. Healy, and Victor L. Bernard: A guide to financial statement analysis.
17.3. Professional Certifications
- Certified Analytics Professional (CAP): A certification for analytics professionals.
- Certified Business Analysis Professional (CBAP): A certification for business analysts.
- Financial Risk Manager (FRM): A certification for risk management professionals.
18. Case Studies in Analysis
Examining real-world case studies can provide valuable insights into how analysis is applied in practice.
18.1. Netflix: Using Data Analysis to Personalize Recommendations
Netflix uses data analysis to understand user preferences and provide personalized recommendations.
- Data Collection: Collecting data on user viewing habits, ratings, and search history.
- Data Analysis: Using machine learning algorithms to identify patterns and relationships in the data.
- Personalized Recommendations: Providing personalized recommendations based on user preferences.
18.2. Amazon: Using Data Analysis to Optimize Supply Chain Management
Amazon uses data analysis to optimize its supply chain management and ensure timely delivery of products.
- Data Collection: Collecting data on inventory levels, shipping times, and customer demand.
- Data Analysis: Using predictive analytics to forecast demand and optimize inventory levels.
- Supply Chain Optimization: Streamlining the supply chain to reduce costs and improve delivery times.
18.3. Google: Using Data Analysis to Improve Search Results
Google uses data analysis to improve its search results and provide users with the most relevant information.
- Data Collection: Collecting data on user search queries, click-through rates, and website content.
- Data Analysis: Using machine learning algorithms to rank search results based on relevance.
- Search Engine Optimization: Improving the relevance and quality of search results.
19. Common Mistakes in Analysis
Even experienced analysts can make mistakes. Being aware of these common pitfalls can help you avoid them.
19.1. Overlooking Assumptions
Failing to question the underlying assumptions of the analysis can lead to flawed conclusions.
- Example: Assuming that correlation implies causation without considering other factors.
19.2. Ignoring Data Quality Issues
Using data that is incomplete, inaccurate, or biased can compromise the integrity of the analysis.
- Example: Not addressing missing values or outliers in the data.
19.3. Overcomplicating the Analysis
Using overly complex methods when simpler techniques would suffice can obscure the results and make them difficult to interpret.
- Example: Applying advanced machine learning algorithms when basic statistical analysis would be more appropriate.
19.4. Misinterpreting Results
Drawing incorrect conclusions from the data can lead to poor decision-making.
- Example: Misinterpreting statistical significance as practical significance.
20. FAQs About What Is An Analysis
Navigating the world of analysis often raises questions. Here are some frequently asked questions to provide clarity.
Question | Answer |
---|---|
What is the primary goal of an analysis? | The primary goal is to transform raw data into actionable intelligence for informed decision-making. |
What are the key components of an analysis? | The key components include data collection, processing, analysis, interpretation, and reporting. |
How does qualitative analysis differ from quantitative analysis? | Qualitative analysis explores non-numerical data to understand meanings, while quantitative analysis uses numerical data and statistical methods to quantify relationships. |
Why is critical thinking important in analysis? | Critical thinking helps evaluate information, identify assumptions, and form well-reasoned conclusions. |
What role does ethics play in analysis? | Ethics ensures that analysis is objective, transparent, and unbiased, protecting data privacy and avoiding misrepresentation. |
How are AI and machine learning changing analysis? | AI and ML are automating tasks, discovering patterns, and making predictions with greater accuracy, transforming data analysis processes. |
What is gap analysis used for? | Gap analysis compares actual performance with desired performance to identify areas for improvement and develop action plans. |
How can I improve my analytical skills? | You can enhance your skills through active listening, effective communication, continuous learning, and attention to detail. |
What tools are commonly used in analysis? | Common tools include spreadsheet software, statistical software, data visualization tools, and database management systems. |
How does risk analysis benefit organizations? | Risk analysis helps identify, assess, and mitigate potential risks, minimizing the likelihood and impact of adverse events. |
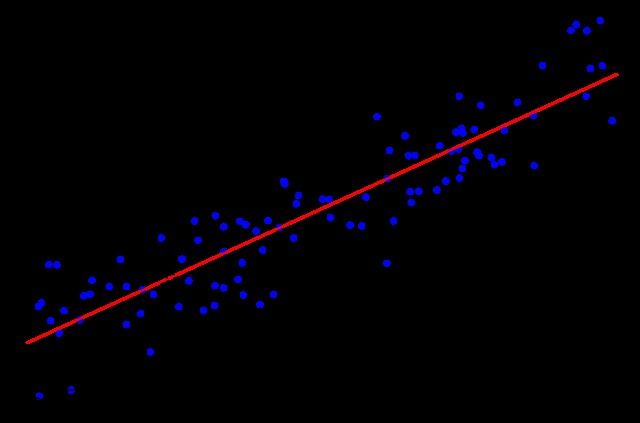
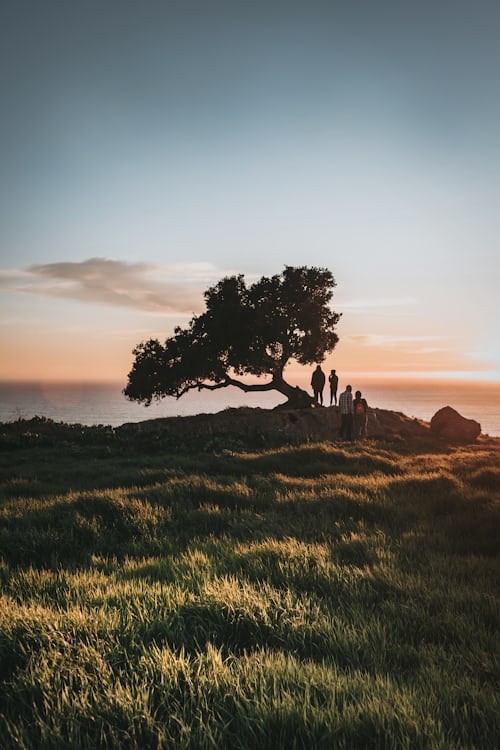
Still have questions? Don’t hesitate to ask on WHAT.EDU.VN! We provide free answers to all your questions and connect you with a community of experts.
Conclusion: Embrace the Power of Analysis
Analysis is a powerful tool that enables us to understand the world around us, solve complex problems, and make informed decisions. By mastering the principles and techniques of analysis, you can unlock new insights and drive positive change in your personal and professional life.
Ready to dive deeper? Visit WHAT.EDU.VN today to ask your questions and receive free, expert answers. Our platform is designed to provide you with the knowledge and support you need to succeed.
Alt text: Person contemplating a complex data chart on a computer screen, symbolizing analytical thinking and decision-making.
Unlock Insights: Ask Your Analysis Questions on WHAT.EDU.VN Today!
Are you struggling to understand a complex topic? Do you need help analyzing data or making informed decisions? Look no further than WHAT.EDU.VN. Our platform provides a free and easy way to ask any question and receive expert answers from our community of knowledgeable users. Whether you’re a student, professional, or simply curious, WHAT.EDU.VN is your go-to resource for all things analysis.
Why Choose WHAT.EDU.VN?
- Free Answers: Get expert answers to your questions without any cost.
- Fast Responses: Receive timely responses from our community of experts.
- Easy to Use: Our platform is intuitive and user-friendly.
- Comprehensive Knowledge Base: Access a wealth of information and resources.
Contact Us
Address: 888 Question City Plaza, Seattle, WA 98101, United States
Whatsapp: +1 (206) 555-7890
Website: WHAT.EDU.VN
Don’t let your questions go unanswered. Visit what.edu.vn today and unlock the power of analysis!