What is the null hypothesis? WHAT.EDU.VN provides a clear explanation. Grasp the concept of no statistical significance with us and explore examples of null hypothesis testing for investment strategies and more. Discover how to confidently use hypothesis testing and statistical analysis. Learn about hypothesis testing, statistical significance, and quantitative analysis.
1. Understanding the Null Hypothesis: The Foundation of Statistical Testing
In statistical analysis, the null hypothesis, often denoted as H0, is a fundamental concept. It proposes that there is no significant difference or relationship between the variables being studied. In simpler terms, it assumes that any observed effect is due to chance or random variation. The null hypothesis serves as a starting point for statistical testing, and researchers aim to either reject or fail to reject it based on the evidence gathered from sample data. This process, known as null hypothesis testing, is crucial for making informed decisions and drawing valid conclusions in various fields, from scientific research to business analytics. Understanding the null hypothesis is essential for anyone seeking to interpret statistical results and make data-driven decisions with confidence.
1.1. What is the Definition of the Null Hypothesis?
The null hypothesis (H0) is a statement that there is no effect or no difference. It’s a starting point for testing a claim or theory. It’s the opposite of what the researcher is trying to prove. Think of it as a “status quo” assumption that needs to be challenged with evidence. For example, if you are testing whether a new drug is effective, the null hypothesis would be that the drug has no effect. The goal of hypothesis testing is to determine whether there is enough evidence to reject the null hypothesis in favor of the alternative hypothesis.
1.2. Why is the Null Hypothesis Important in Statistical Testing?
The null hypothesis is important because it provides a framework for making decisions based on data. It allows researchers to quantify the evidence against a specific claim. By setting up a null hypothesis, researchers can determine the probability of observing the data if the null hypothesis were true. This probability, known as the p-value, helps researchers decide whether to reject the null hypothesis. Without a null hypothesis, it would be difficult to objectively evaluate the evidence and draw meaningful conclusions.
1.3. What are the Key Characteristics of a Good Null Hypothesis?
A good null hypothesis should be:
- Testable: It should be possible to collect data and perform statistical tests to evaluate the hypothesis.
- Specific: It should clearly state the expected outcome if there is no effect or no difference.
- Falsifiable: It should be possible to reject the null hypothesis if there is enough evidence against it.
- Simple: It should be as simple as possible while still addressing the research question.
2. Deconstructing the Null Hypothesis: A Closer Look at its Components
To truly understand the null hypothesis, it’s crucial to break down its components. This involves identifying the variables being studied, the population of interest, and the specific statement being made about the relationship between these elements. For instance, in a study comparing the effectiveness of two different teaching methods, the null hypothesis might state that there is no difference in the average test scores of students taught using either method. This statement clearly defines the variables (teaching method and test scores), the population (students), and the expected outcome (no difference in scores). By carefully deconstructing the null hypothesis, researchers can ensure that their statistical tests are appropriately designed and that their conclusions are valid.
2.1. Identifying Variables and Parameters in a Null Hypothesis
Identifying variables and parameters is a crucial step in formulating a null hypothesis. Variables are the characteristics or attributes that are being measured or observed in a study. Parameters are numerical values that describe a population, such as the mean or standard deviation. The null hypothesis typically makes a statement about the value of a population parameter. For example, if you are testing whether the average height of women is 5’4″, the variable is height, and the parameter is the population mean height of women. The null hypothesis would be that the population mean height of women is equal to 5’4″.
2.2. Understanding the Difference Between a Null and Alternative Hypothesis
The null hypothesis (H0) and the alternative hypothesis (H1 or Ha) are two mutually exclusive statements about a population. The null hypothesis states that there is no effect or no difference, while the alternative hypothesis states that there is an effect or a difference. In other words, the alternative hypothesis is the opposite of the null hypothesis. For example, if the null hypothesis is that a new drug has no effect, the alternative hypothesis would be that the drug does have an effect. Statistical tests are used to determine whether there is enough evidence to reject the null hypothesis in favor of the alternative hypothesis.
2.3. Recognizing Common Types of Null Hypotheses
There are several common types of null hypotheses, including:
- Hypothesis of no difference: This type of hypothesis states that there is no difference between two groups or populations.
- Hypothesis of no association: This type of hypothesis states that there is no relationship between two variables.
- Hypothesis of no effect: This type of hypothesis states that a treatment or intervention has no effect on an outcome.
- Hypothesis of equality: This type of hypothesis states that two population parameters are equal.
3. Crafting a Null Hypothesis: A Step-by-Step Guide
Creating a clear and testable null hypothesis is a critical step in any statistical investigation. This process involves several key steps: first, clearly define the research question you are trying to answer. Then, identify the variables you will be studying and the population you are interested in. Next, formulate a statement that assumes there is no relationship or difference between the variables in the population. This statement should be specific and measurable, allowing you to collect data and perform statistical tests to evaluate its validity. Finally, ensure that your null hypothesis is falsifiable, meaning that it is possible to reject it if the evidence suggests otherwise. By following these steps, you can craft a null hypothesis that is both meaningful and statistically sound.
3.1. Defining Your Research Question Clearly
Before you can formulate a null hypothesis, you need to have a clear research question. Your research question should be specific, focused, and answerable. It should also be relevant to your field of study and contribute to the existing body of knowledge. For example, a research question might be: “Does a new teaching method improve student test scores?” or “Is there a relationship between exercise and weight loss?”
3.2. Identifying the Variables and Population of Interest
Once you have a clear research question, you need to identify the variables you will be studying and the population you are interested in. Variables are the characteristics or attributes that you will be measuring or observing. The population is the entire group of individuals or objects that you are interested in studying. For example, in the research question “Does a new teaching method improve student test scores?”, the variables are teaching method and test scores, and the population is all students who could potentially be taught using the new method.
3.3. Formulating a Statement of No Effect or No Difference
The null hypothesis is a statement of no effect or no difference. It states that there is no relationship between the variables you are studying, or that there is no difference between two groups or populations. The null hypothesis should be specific and measurable. It should also be falsifiable, meaning that it is possible to reject the null hypothesis if there is enough evidence against it. For example, in the research question “Does a new teaching method improve student test scores?”, the null hypothesis might be: “There is no difference in average test scores between students taught using the new teaching method and students taught using the traditional teaching method.”
3.4. Ensuring Your Null Hypothesis is Falsifiable
A falsifiable null hypothesis is one that can be proven wrong through experimentation or observation. It’s a critical aspect of the scientific method because it allows researchers to test their ideas and potentially revise them based on evidence. If a null hypothesis cannot be proven wrong, it’s not a useful hypothesis because it doesn’t allow for the possibility of learning something new. To ensure your null hypothesis is falsifiable, make sure it makes a specific claim that can be tested with data.
4. Examples of Null Hypotheses Across Different Fields
The null hypothesis is a versatile tool used in various disciplines to test specific claims or theories. In the medical field, a null hypothesis might state that a new drug has no effect on a particular disease. In marketing, it could propose that there is no difference in sales between two different advertising campaigns. In education, a null hypothesis might suggest that a new teaching method does not improve student performance. These examples illustrate how the null hypothesis provides a starting point for statistical testing, allowing researchers to gather evidence and determine whether there is enough support to reject the assumption of no effect or no difference.
4.1. Null Hypotheses in Medical Research
In medical research, null hypotheses are commonly used to test the effectiveness of new treatments or interventions. For example, a researcher might formulate the null hypothesis that a new drug has no effect on blood pressure. This hypothesis would be tested by comparing the blood pressure of patients who receive the drug to the blood pressure of patients who receive a placebo. If the data show a statistically significant difference in blood pressure between the two groups, the researcher would reject the null hypothesis and conclude that the drug does have an effect on blood pressure.
4.2. Null Hypotheses in Business and Marketing
In business and marketing, null hypotheses are often used to test the effectiveness of different marketing strategies or business practices. For example, a marketing manager might formulate the null hypothesis that there is no difference in sales between two different advertising campaigns. This hypothesis would be tested by comparing the sales generated by each campaign. If the data show a statistically significant difference in sales between the two campaigns, the marketing manager would reject the null hypothesis and conclude that one campaign is more effective than the other.
4.3. Null Hypotheses in Social Sciences
In the social sciences, null hypotheses are used to test theories about human behavior and social phenomena. For example, a sociologist might formulate the null hypothesis that there is no relationship between education level and income. This hypothesis would be tested by analyzing data on education levels and incomes. If the data show a statistically significant relationship between the two variables, the sociologist would reject the null hypothesis and conclude that there is a relationship between education level and income.
5. Testing the Null Hypothesis: The Process of Statistical Inference
Testing the null hypothesis is the core of statistical inference. This process involves collecting data, calculating relevant statistics, and determining the probability of observing the data if the null hypothesis were true. This probability is known as the p-value. If the p-value is below a predetermined significance level (alpha), typically 0.05, the null hypothesis is rejected. This means that the evidence suggests there is a statistically significant effect or difference. Conversely, if the p-value is above alpha, the null hypothesis is not rejected, indicating that the evidence is not strong enough to support the alternative hypothesis. Understanding this process is crucial for interpreting statistical results and drawing valid conclusions from data.
5.1. Understanding P-Values and Significance Levels
P-values and significance levels are essential concepts in hypothesis testing. The p-value is the probability of observing data as extreme as, or more extreme than, the data actually observed, assuming that the null hypothesis is true. In other words, it’s a measure of how likely the observed results are if there is truly no effect or no difference. The significance level (alpha) is a predetermined threshold that is used to decide whether to reject the null hypothesis. Typically, a significance level of 0.05 is used, which means that there is a 5% chance of rejecting the null hypothesis when it is actually true (Type I error). If the p-value is less than or equal to the significance level, the null hypothesis is rejected.
5.2. Choosing the Right Statistical Test for Your Null Hypothesis
Choosing the right statistical test is crucial for accurately testing your null hypothesis. The choice of test depends on several factors, including the type of data you have (e.g., categorical or continuous), the number of groups you are comparing, and the nature of the relationship you are investigating. Some common statistical tests include t-tests, ANOVA, chi-square tests, and correlation analysis. Consulting with a statistician or using a statistical software package can help you determine the appropriate test for your specific research question and data.
5.3. Interpreting the Results: Rejecting or Failing to Reject the Null Hypothesis
Interpreting the results of a hypothesis test involves deciding whether to reject or fail to reject the null hypothesis. If the p-value is less than or equal to the significance level, you reject the null hypothesis. This means that there is enough evidence to conclude that there is a statistically significant effect or difference. If the p-value is greater than the significance level, you fail to reject the null hypothesis. This means that there is not enough evidence to conclude that there is a statistically significant effect or difference. It’s important to note that failing to reject the null hypothesis does not mean that the null hypothesis is true. It simply means that there is not enough evidence to reject it.
6. Common Mistakes to Avoid When Working with Null Hypotheses
Working with null hypotheses can be tricky, and it’s easy to make mistakes that can lead to incorrect conclusions. One common mistake is failing to clearly define the null hypothesis before conducting the study. Another is choosing the wrong statistical test, which can lead to inaccurate p-values and incorrect decisions. A third mistake is misinterpreting the results, such as assuming that failing to reject the null hypothesis means it is true. To avoid these pitfalls, it’s essential to carefully plan your study, choose the appropriate statistical tests, and interpret the results cautiously, considering the limitations of your data and analysis.
6.1. Misinterpreting “Failure to Reject” as “Acceptance”
One of the most common mistakes in hypothesis testing is misinterpreting “failure to reject” the null hypothesis as “acceptance” of the null hypothesis. When you fail to reject the null hypothesis, it simply means that there is not enough evidence to conclude that the null hypothesis is false. It does not mean that the null hypothesis is true. There may be other factors at play, or the sample size may not be large enough to detect a significant effect.
6.2. Choosing the Wrong Statistical Test
Choosing the wrong statistical test can lead to incorrect conclusions. The choice of statistical test depends on the type of data you have, the number of groups you are comparing, and the nature of the relationship you are investigating. Using the wrong test can lead to inaccurate p-values and incorrect decisions about whether to reject the null hypothesis. It’s important to carefully consider the assumptions of each statistical test and choose the test that is most appropriate for your data.
6.3. Ignoring the Assumptions of Statistical Tests
Statistical tests are based on certain assumptions about the data. If these assumptions are not met, the results of the test may be invalid. Some common assumptions include normality, independence, and homogeneity of variance. It’s important to check whether these assumptions are met before conducting a statistical test. If the assumptions are violated, you may need to use a different test or transform your data.
7. The Role of Sample Size in Null Hypothesis Testing
Sample size plays a critical role in null hypothesis testing. A larger sample size increases the power of the test, making it more likely to detect a true effect if one exists. With a small sample size, even a substantial effect may not be statistically significant, leading to a failure to reject the null hypothesis. Conversely, a very large sample size can lead to statistically significant results even for small, practically insignificant effects. Therefore, it’s essential to carefully consider the sample size when designing a study and interpreting the results of hypothesis testing.
7.1. How Sample Size Affects the Power of a Statistical Test
The power of a statistical test is the probability of rejecting the null hypothesis when it is false. In other words, it’s the ability of the test to detect a true effect. Sample size is a major determinant of power. As sample size increases, the power of the test also increases. This is because larger samples provide more information about the population, which reduces the variability in the data and makes it easier to detect a significant effect.
7.2. Determining an Appropriate Sample Size for Your Study
Determining an appropriate sample size is crucial for ensuring that your study has enough power to detect a true effect. There are several methods for determining sample size, including power analysis, which involves estimating the sample size needed to achieve a desired level of power. Other methods include using rules of thumb or consulting with a statistician. The appropriate sample size will depend on several factors, including the size of the effect you are trying to detect, the variability in the data, and the desired level of power.
7.3. The Impact of Large Sample Sizes on Statistical Significance
While larger sample sizes generally increase the power of a statistical test, they can also lead to statistically significant results even for small, practically insignificant effects. This is because larger samples reduce the variability in the data, making it easier to detect even tiny differences. Therefore, it’s important to consider the practical significance of the results, in addition to the statistical significance, when interpreting the results of hypothesis testing with large sample sizes.
8. Type I and Type II Errors: Understanding the Risks in Hypothesis Testing
In hypothesis testing, there are two types of errors that can occur: Type I and Type II errors. A Type I error, also known as a false positive, occurs when the null hypothesis is rejected when it is actually true. A Type II error, also known as a false negative, occurs when the null hypothesis is not rejected when it is actually false. Understanding these errors is crucial for interpreting the results of hypothesis testing and making informed decisions based on the evidence.
8.1. Defining Type I and Type II Errors
- Type I Error (False Positive): Rejecting the null hypothesis when it is actually true.
- Type II Error (False Negative): Failing to reject the null hypothesis when it is actually false.
8.2. The Consequences of Each Type of Error
The consequences of each type of error depend on the context of the research. In medical research, a Type I error could lead to the approval of an ineffective drug, while a Type II error could lead to the rejection of a potentially life-saving treatment. In business, a Type I error could lead to the implementation of a flawed marketing strategy, while a Type II error could lead to the missed opportunity to capitalize on a successful product.
8.3. Balancing the Risk of Type I and Type II Errors
Balancing the risk of Type I and Type II errors involves choosing an appropriate significance level (alpha) and ensuring that the study has enough power. A lower significance level reduces the risk of a Type I error but increases the risk of a Type II error. Increasing the sample size increases the power of the study and reduces the risk of a Type II error. The optimal balance between the two types of errors depends on the specific research question and the consequences of each type of error.
9. Null Hypothesis Testing in the Age of Big Data
The rise of big data has brought new challenges and opportunities to null hypothesis testing. With massive datasets, even small effects can become statistically significant, raising concerns about the practical relevance of the findings. Furthermore, the sheer volume of data can lead to spurious correlations and an increased risk of Type I errors. To address these challenges, researchers are developing new methods for hypothesis testing that are better suited for big data, such as adjusting significance levels and focusing on effect sizes rather than just p-values.
9.1. The Challenges of Applying Null Hypothesis Testing to Large Datasets
Applying null hypothesis testing to large datasets presents several challenges:
- Statistical Significance vs. Practical Significance: With large datasets, even small effects can become statistically significant, but they may not be practically meaningful.
- Spurious Correlations: Large datasets increase the risk of finding spurious correlations, which are relationships that appear to be statistically significant but are actually due to chance.
- Increased Risk of Type I Errors: The more tests you perform, the greater the chance of making a Type I error.
9.2. Alternative Approaches to Hypothesis Testing in the Era of Big Data
To address the challenges of hypothesis testing in the era of big data, researchers are exploring alternative approaches:
- Focusing on Effect Sizes: Instead of relying solely on p-values, researchers are focusing on effect sizes, which measure the magnitude of the effect.
- Adjusting Significance Levels: Researchers are adjusting significance levels to account for the increased risk of Type I errors when performing multiple tests.
- Using Bayesian Methods: Bayesian methods provide a framework for incorporating prior knowledge into the analysis, which can help to reduce the risk of spurious findings.
9.3. The Future of Statistical Inference in a Data-Driven World
The future of statistical inference in a data-driven world will likely involve a combination of traditional hypothesis testing methods and new approaches that are better suited for big data. Researchers will need to be more aware of the limitations of traditional methods and be willing to adopt new techniques that can help them to draw more meaningful conclusions from large datasets.
10. Seeking Help with Null Hypothesis and Statistical Questions: WHAT.EDU.VN is Here to Assist
Navigating the complexities of null hypothesis testing and statistical analysis can be daunting. Many individuals, from students to professionals, find themselves struggling to grasp these concepts and apply them effectively. Whether you’re facing difficulties with homework assignments, research projects, or data-driven decision-making, seeking help from a reliable source can make a significant difference.
10.1. The Convenience and Accessibility of Online Question-Answering Platforms
Online question-answering platforms offer a convenient and accessible way to get help with null hypothesis and statistical questions. These platforms provide a forum for asking questions and receiving answers from experts and peers. This can be especially helpful for students who are struggling with their coursework or professionals who need assistance with data analysis.
10.2. The Benefits of Free Consultation Services
Free consultation services offer a valuable opportunity to get personalized help with null hypothesis and statistical questions. These services typically involve a one-on-one consultation with an expert who can provide guidance and support. This can be especially helpful for individuals who have complex questions or who need help with a specific research project.
10.3. WHAT.EDU.VN: Your Go-To Resource for Statistical Support
At WHAT.EDU.VN, we understand the challenges of working with null hypotheses and statistical concepts. That’s why we offer a comprehensive platform designed to provide you with the support you need to succeed. Whether you’re looking for quick answers to specific questions or in-depth guidance on complex statistical analyses, our team of experts is here to assist you.
- Ask any question: Our platform allows you to ask any question related to null hypothesis testing, statistical analysis, or any other topic.
- Get answers fast: Our team of experts provides prompt and accurate answers to your questions.
- Receive free consultations: We offer free consultation services to help you with your specific research projects or data analysis needs.
Don’t let statistical challenges hold you back. Visit WHAT.EDU.VN today and experience the convenience and support of our comprehensive question-answering platform.
We are located at 888 Question City Plaza, Seattle, WA 98101, United States. You can also reach us via Whatsapp at +1 (206) 555-7890. Visit our website at WHAT.EDU.VN to learn more.
Why struggle with statistical concepts when you can get the help you need quickly and easily? Visit WHAT.EDU.VN now and ask your question. Get the answers you need to succeed!
FAQ: Null Hypothesis
Question | Answer |
---|---|
What is a null hypothesis in simple terms? | The null hypothesis states there is no significant difference or relationship between variables. It assumes any observed effect is due to chance. |
How do I formulate a null hypothesis? | 1. Define your research question. 2. Identify variables and population. 3. Formulate a statement assuming no effect or difference. 4. Ensure it’s falsifiable. |
What is the difference between a null and an alternative hypothesis? | The null hypothesis states no effect or difference, while the alternative hypothesis states there is an effect or difference. They are mutually exclusive. |
What is a p-value, and how does it relate to the null hypothesis? | The p-value is the probability of observing data as extreme as, or more extreme than, the data observed, assuming the null hypothesis is true. If the p-value is below the significance level (alpha), you reject the null hypothesis. |
What is a Type I error? | A Type I error (false positive) occurs when you reject the null hypothesis when it is actually true. |
What is a Type II error? | A Type II error (false negative) occurs when you fail to reject the null hypothesis when it is actually false. |
How does sample size affect hypothesis testing? | Larger sample sizes increase the power of the test, making it more likely to detect a true effect. However, very large samples can lead to statistically significant results even for small, practically insignificant effects. |
What are some common mistakes to avoid? | Misinterpreting “failure to reject” as “acceptance,” choosing the wrong statistical test, and ignoring the assumptions of statistical tests are common mistakes. |
How has big data impacted null hypothesis testing? | Big data presents challenges such as spurious correlations and increased risk of Type I errors. Alternative approaches include focusing on effect sizes and adjusting significance levels. |
Where can I get help with null hypothesis and statistical questions? | Platforms like what.edu.vn offer convenient question-answering services, free consultations, and expert support to help you navigate statistical challenges. |
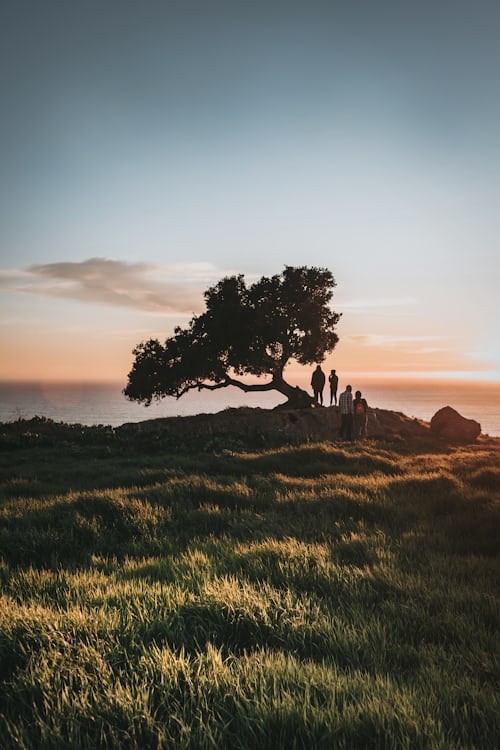
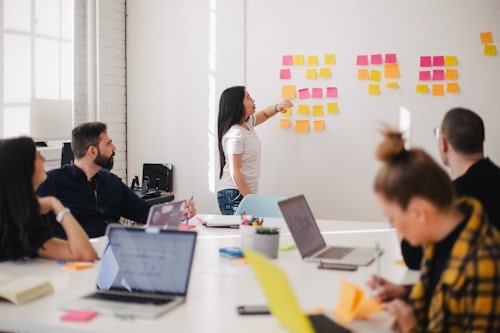